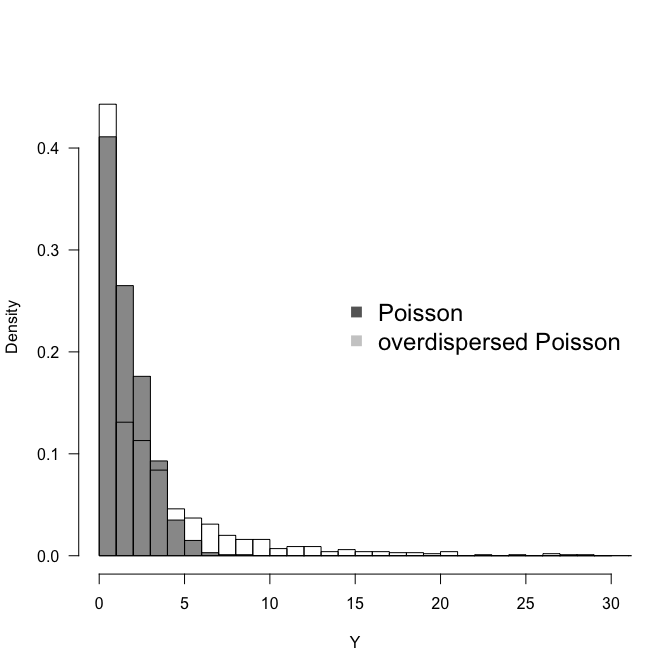
Overdispersion
In statistics, overdispersion is the presence of greater variability (statistical dispersion) in a data set than would be expected based on a given statistical model. Overdispersion is often encountered when fitting very simple parametric models, such as those based on the Poisson distribution and this will usually lead to inflated Type I error rates. The Poisson distribution has one free parameter and does not allow for the variance to be adjusted independently of the mean. The choice of a distribution from the Poisson family is often dictated by the nature of the empirical data. For example, Poisson regression analysis is commonly used to model count data. If overdispersion is a feature, an alternative model with additional free parameters may provide a better fit. In the case of count data, a Poisson mixture model like the negative binomial distribution can be proposed instead, in which the mean of the Poisson distribution can itself be thought of as a random variable drawn – in this case – from the gamma distribution thereby introducing an additional free parameter (note the resulting negative binomial distribution is completely characterized by two parameters).
Example: Extramarital Affairs
Description: Data set includes various social and marital factors along with the count of extramarital affairs for 600 people.
naffairs: Number of affairs within last year.
kids: 1=have children 0=no children.
vryunhap: Very unhappily married (1/0).
unhap: Unhappily married (1/0).
avgmarr: Average married (1/0).
hapavg: Happily married (1.0).
vryhap: Very happily married (1/0).
antirel: Anti-religious (1/0)
notrel: Not religious (1/0).
slghtrel: Slightly religious (1/0).
smerel: Somewhat religious (1/0).
vryrel: Very religious (1/0).
yrsmarr1: Year of marriage > 0.75 yrs (1/0).
yrsmarr2: Year of marriage > 1.5 yrs (1/0).
yrsmarr3: Year of marriage > 4.0 yrs (1/0).
yrsmarr4: Year of marriage > 7.0 yrs (1/0).
yrsmarr5: Year of marriage > 10.0 yrs (1/0).
yrsmarr6: Year of marriage > 15.0 yrs (1/0).
Source: https://vincentarelbundock.github.io/Rdatasets/doc/COUNT/affairs.html
Download the data from here
Task: Design a model for estimating number of affairs.
Let's try PROC GENMOD with poisson distribution option:
PROC GENMOD DATA=tutorial.affairs;
CLASS kids--yrsmarr6;
MODEL naffairs = kids--yrsmarr6 / DIST=POISSON;
RUN;
Criteria For Assessing Goodness Of Fit | |||
---|---|---|---|
Criterion | DF | Value | Value/DF |
Deviance | 586 | 2303.1626 | 3.9303 |
Scaled Deviance | 586 | 2303.1626 | 3.9303 |
Pearson Chi-Square | 586 | 4050.5305 | 6.9122 |
Scaled Pearson X2 | 586 | 4050.5305 | 6.9122 |
Log Likelihood | -235.1784 | ||
Full Log Likelihood | -1398.5763 | ||
AIC (smaller is better) | 2827.1525 | ||
AICC (smaller is better) | 2827.9730 | ||
BIC (smaller is better) | 2893.1314 |
‘Criteria For Assessing Goodness Of Fit’ section of output suggests that, because value/df for both deviance and Pearson Chi-Square statistics is much higher than 1, Poisson model is not quite adequate to describe the counts of hypotensions and crampings. It also suggests that there is overdispersion.
Negative-binomial distribution is a benchmark model to account for overdisperion on count responses. We can test for overdispersion in a Poisson model by using the DIST=NEGBIN, SCALE=0, and NOSCALE options in the MODEL statement of PROC GENMOD. When used together, these options test whether overdispersion of the form μ+kμ2 exists by testing whether the negative binomial dispersion parameter, k, is zero. When k=0, the negative binomial distribution is equivalent to the Poisson distribution. Let's test whether there is overdispersion in our data.
PROC GENMOD DATA=tutorial.affairs;
CLASS kids--yrsmarr6;
MODEL naffairs = kids--yrsmarr6 / DIST=NEGBIN SCALE=0 NOSCALE;
RUN;
Lagrange Multiplier Statistics | |||
---|---|---|---|
Parameter | Chi-Square | Pr > ChiSq | |
Dispersion | 259.7461 | <.0001 | * |
* One-sided p-value |
Our suspicions were correct; there is overdispersion. There are various distributions to implement instead of Poisson:
- Negative binomial
- Zero-inflated poisson
- Zero-inflated negative binomial
- Hurdle poisson
- Hurdle negative binomial
- Generalized poisson
PROC GENMOD DATA=tutorial.affairs;
CLASS kids--yrsmarr6;
MODEL naffairs = kids--yrsmarr6 / DIST=POISSON DSCALE;
RUN;
Criteria For Assessing Goodness Of Fit | |||
---|---|---|---|
Criterion | DF | Value | Value/DF |
Deviance | 586 | 2303.1626 | 3.9303 |
Scaled Deviance | 586 | 586.000 | 1.000 |
Pearson Chi-Square | 586 | 4050.5305 | 6.9122 |
Scaled Pearson X2 | 586 | 1030.5876 | 1.7587 |
Log Likelihood | -59.8371 | ||
Full Log Likelihood | -1398.5763 | ||
AIC (smaller is better) | 2827.1525 | ||
AICC (smaller is better) | 2827.9730 | ||
BIC (smaller is better) | 2893.1314 |
Scaled Pearson X2 is still well above 1, therefore we will try negative binomial distribution next:
PROC GENMOD DATA=tutorial.affairs;
CLASS kids--yrsmarr6;
MODEL naffairs = kids--yrsmarr6 / DIST=NEGBIN;
RUN;
Criteria For Assessing Goodness Of Fit | |||
---|---|---|---|
Criterion | DF | Value | Value/DF |
Deviance | 586 | 339.8629 | 0.5800 |
Scaled Deviance | 586 | 339.8629 | 0.5800 |
Pearson Chi-Square | 586 | 566.8541 | 0.9673 |
Scaled Pearson X2 | 586 | 566.8541 | 0.9673 |
Log Likelihood | 439.3546 | ||
Full Log Likelihood | -724.0433 | ||
AIC (smaller is better) | 1480.0865 | ||
AICC (smaller is better) | 1481.0180 | ||
BIC (smaller is better) | 1550.4640 |
Parameter estimates (not shown) tells us that only unhap, antirel, notrel, slghtrel, yrsmarr1, yrsmarr2, yrsmarr3 are significant. We run our model with only these variables:
PROC GENMOD DATA=tutorial.affairs;
CLASS kids--yrsmarr6;
MODEL naffairs = unhap antirel notrel slghtrel yrsmarr1 yrsmarr2 yrsmarr3 / DIST=NEGBIN;
RUN;
Criteria For Assessing Goodness Of Fit | |||
---|---|---|---|
Criterion | DF | Value | Value/DF |
Deviance | 593 | 338.5604 | 0.5709 |
Scaled Deviance | 593 | 338.5604 | 0.5709 |
Pearson Chi-Square | 593 | 530.2979 | 0.8943 |
Scaled Pearson X2 | 593 | 530.2979 | 0.8943 |
Log Likelihood | 435.7320 | ||
Full Log Likelihood | -727.6659 | ||
AIC (smaller is better) | 1473.3317 | ||
AICC (smaller is better) | 1473.6363 | ||
BIC (smaller is better) | 1512.9191 |
Algorithm converged. |
Analysis Of Maximum Likelihood Parameter Estimates | ||||||||
---|---|---|---|---|---|---|---|---|
Parameter | DF | Estimate | Standard Error |
Wald 95% Confidence Limits | Wald Chi-Square | Pr > ChiSq | ||
Intercept | 1 | 0.6383 | 0.9363 | -1.1968 | 2.4735 | 0.46 | 0.4954 | |
unhap | 0 | 1 | -1.0441 | 0.3624 | -1.7544 | -0.3339 | 8.30 | 0.0040 |
antirel | 0 | 1 | -1.3962 | 0.4489 | -2.2760 | -0.5164 | 9.67 | 0.0019 |
notrel | 0 | 1 | -1.1257 | 0.3141 | -1.7413 | -0.5101 | 12.85 | 0.0003 |
slghtrel | 0 | 1 | -1.2156 | 0.3302 | -1.8629 | -0.5683 | 13.55 | 0.0002 |
yrsmarr1 | 0 | 1 | 1.3507 | 0.4769 | 0.4160 | 2.2853 | 8.02 | 0.0046 |
yrsmarr2 | 0 | 1 | 1.6580 | 0.3850 | 0.9034 | 2.4126 | 18.54 | <.0001 |
yrsmarr3 | 0 | 1 | 0.8858 | 0.3404 | 0.2187 | 1.5529 | 6.77 | 0.0093 |
Dispersion | 1 | 7.0132 | 0.7824 | 5.6358 | 8.7272 |
Let's also use SAS graphics to see some of the effects of these parameters. Prior to this we need to reformat the data set and create new columns:
DATA DATA=tutorial.affairs;
SET tutorial.affairs;
IF vryunhap = 1 THEN happiness = 'Very unhappy';
ELSE IF unhap = 1 THEN happiness = 'Unhappy';
ELSE IF hapavg = 1 THEN happiness = 'Happy';
ELSE IF vryhap = 1 THEN happiness = 'Very happy';
ELSE IF avgmarr = 1 THEN happiness = 'Very unhappy';
IF antirel = 1 THEN religiosity = 'Anti religious';
ELSE IF notrel = 1 THEN religiosity = 'Not religious';
ELSE IF slghtrel = 1 THEN religiosity = 'Slightly religious';
ELSE IF smerel = 1 THEN religiosity = 'Somewhat religious';
ELSE IF vryrel = 1 THEN religiosity = 'Very religious';
IF yrsmarr1 = 1 THEN years = '0.75';
ELSE IF yrsmarr2 = 1 THEN years = '1.5';
ELSE IF yrsmarr3 = 1 THEN years = '4';
ELSE IF yrsmarr4 = 1 THEN years = '7';
ELSE IF yrsmarr5 = 1 THEN years = '10';
ELSE IF yrsmarr6 = 1 THEN years = '15';
cnt=1;
RUN;
Now let's use a few graphs to understand these variables:
PROC SGPLOT DATA=tutorial.affairs;
VBAR religiosity / RESPONSE=cnt STATSUM;
XAXIS VALUES =('Anti religious' 'Not religious' 'Slightly religious' 'Somewhat religious' 'Very religious');
RUN;
PROC SGPLOT DATA=tutorial.affairs;
VBAR happiness / RESPONSE=cnt STATSUM;
XAXIS VALUES =('Very unhappy' 'Unhappy' 'Average' 'Happy' 'Very happy');
RUN;
PROC SGPLOT DATA=tutorial.affairs;
VBAR years / RESPONSE=cnt STATSUM;
XAXIS VALUES =('0.75' '1.5' '4' '7' '10' '15');
RUN;
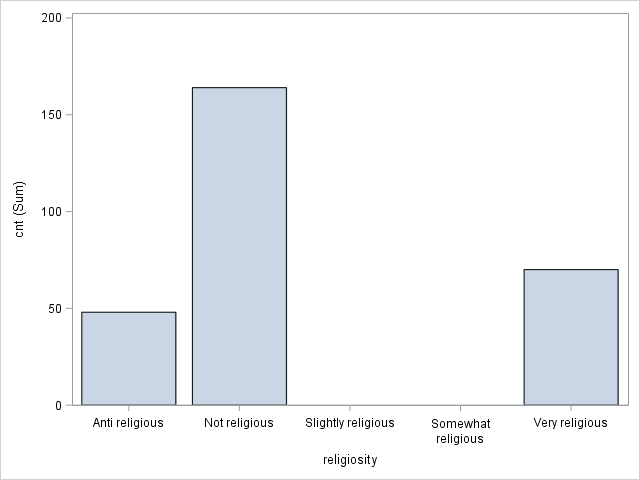
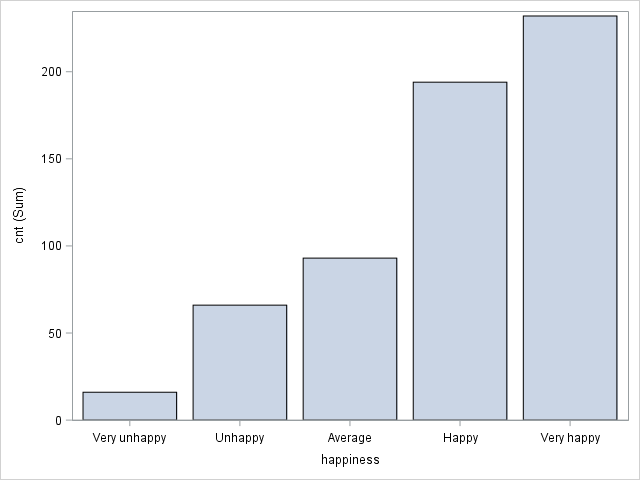
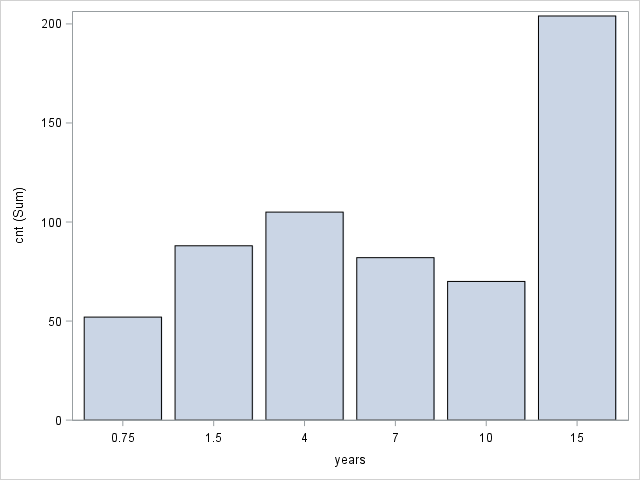
Leave a Comment